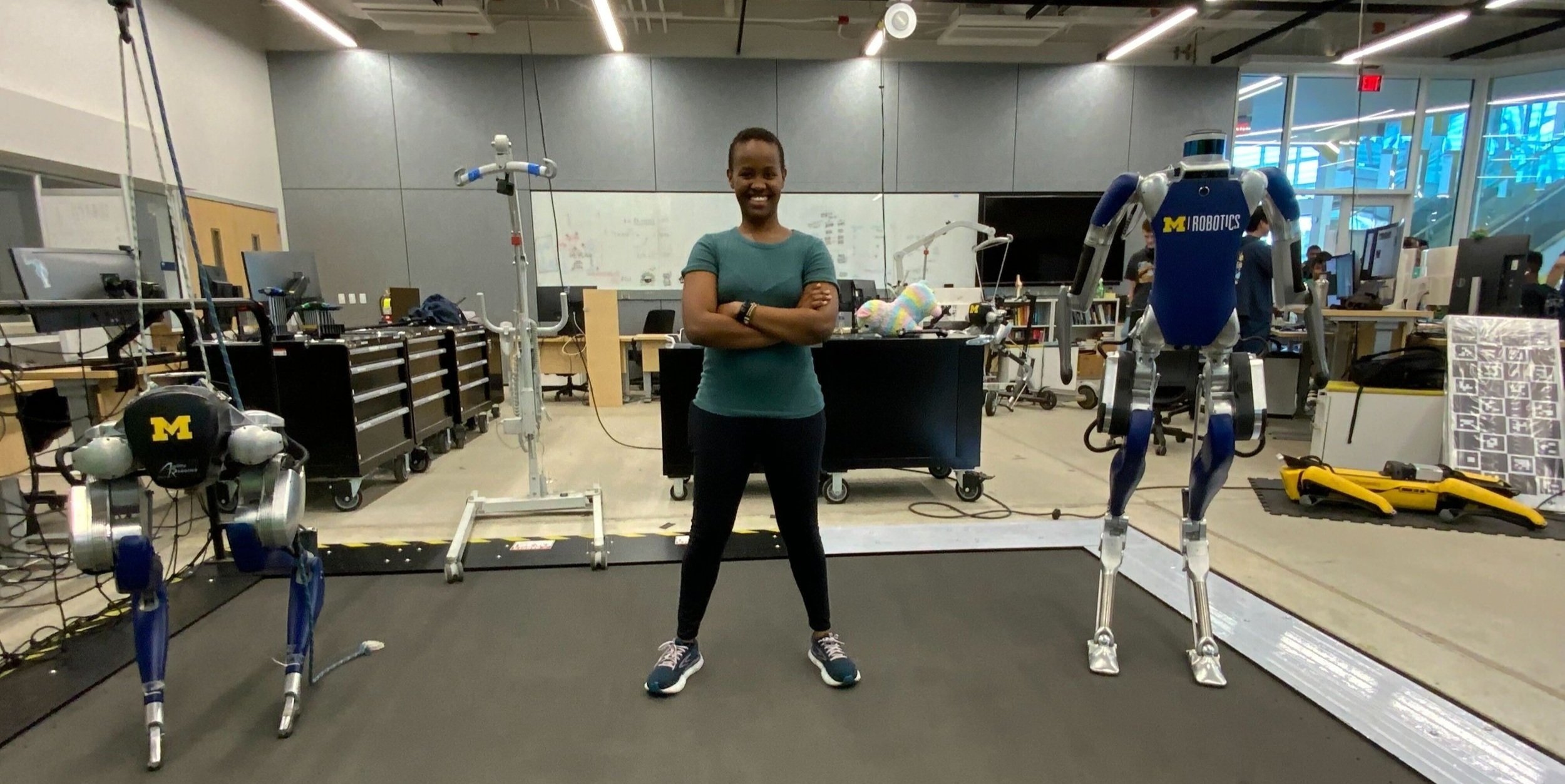
Eva Mungai is Defending!
Eva Mungai is Defending!
Defense Information
I am excited to share that I will be defending my Ph.D. on January 26th at 2 PM EST. The title of my dissertation is: Towards a Fall Tolerant Framework for Bipedal Robots. For more information, please see the abstract.
It would be an honor to have you join me for this significant milestone in my academic journey. You are welcome to attend either in person at the Ford Robotics Building Room 2300 or virtually via zoom.
To join the zoom session, please use the link provided below. Alternatively, feel free to add the event to your calendar for easy access.
-
This dissertation focuses on developing a fall-tolerant framework for bipedal robots, aiming to enhance their ability to navigate challenging situations by effectively assessing, adapting, and responding to uncertainties and disturbances. Bipedal robots, with their unique capability to navigate diverse terrains and restore mobility, are ideal for assisting in critical and day-to-day tasks. However, their real-world deployment is limited due to factors like high-dimensional complex dynamics and a smaller support polygon, making it difficult to achieve stable motion, especially in the face of disturbances and uncertainties.
The dissertation addresses these limitations by developing robust controllers and reliable fall prediction algorithms. Feedback controllers have been used in the literature to ensure robustness against disturbances and uncertainties. However, it is infeasible to account for all disturbances and uncertainties, making falls inevitable. Falls are undesirable as they can prevent a robot from completing its task, result in damage to the surrounding area, or lead to injuries. Therefore, the dissertation emphasizes the importance of implementing robust controllers and employing methods to predict falls.
This research begins by introducing a systematic method to design control objectives for highly constrained systems and concludes by presenting a 1D convolutional neural network fall prediction algorithm capable of not only predicting falls but also estimating the time to react. The effectiveness of the control objectives is demonstrated through robust, comfortable closed-loop sit-to-stand motions for a fully actuated lower-limb exoskeleton, Atalante. The performance of the proposed fall prediction algorithms is evaluated both in simulation using a planar-four link robot based on Atalante and in hardware and simulation for the bipedal robot Digit.
Thank you all!
My PhD journey has been lengthy, challenging, yet deeply rewarding, and I couldn't have done it alone. From Nairobi, California, New York, and finally to Michigan, this voyage has been marked by the unwavering support of various individuals. The saying that it takes a village to raise a child could not be truer. I am genuinely thankful to each person who has supported, encouraged, and believed in me along the way. Thengiu muno!